Thinking About Equity and Bias in AI
Addressing inequity in AI requires an understanding of how bias manifests itself in both society and algorithms.
Your content has been saved!
Go to My Saved Content.It is crucial to be aware of the biases that exist within ourselves and our institutions when attempting to address bias in data and algorithms. While we may be able to mitigate some of the biased outputs that result from these biases, it is more important to understand the root of the problem in order to truly eradicate it. This means acknowledging and understanding the biases that exist within ourselves, our institutions, and the data that we use.
Our technology often reflects the prejudices that exist in society, and it is important to be aware of this so that we can work to create a more equitable world. Transparency in data sourcing and algorithm development, along with the implementation of checks and balances, can help to reduce the risk of biased outputs from AI. However, it is important to remember that we cannot truly address bias without understanding how our own biases contribute to the problem. With humility, it is imperative we acknowledge our part of the problem.
Before we can even start spotting those biases in AI, which requires due diligence and intentionality, we have to get honest with ourselves. This leads us to utilizing a framework from Demarginalizing Design that can be remembered by the mnemonic device, “Am I Right?”:
- Avoiding objective facts.
- Misinterpreting information in a way that only supports existing beliefs.
- Ignoring information that challenges existing beliefs.
- Remembering details that only uphold existing beliefs.
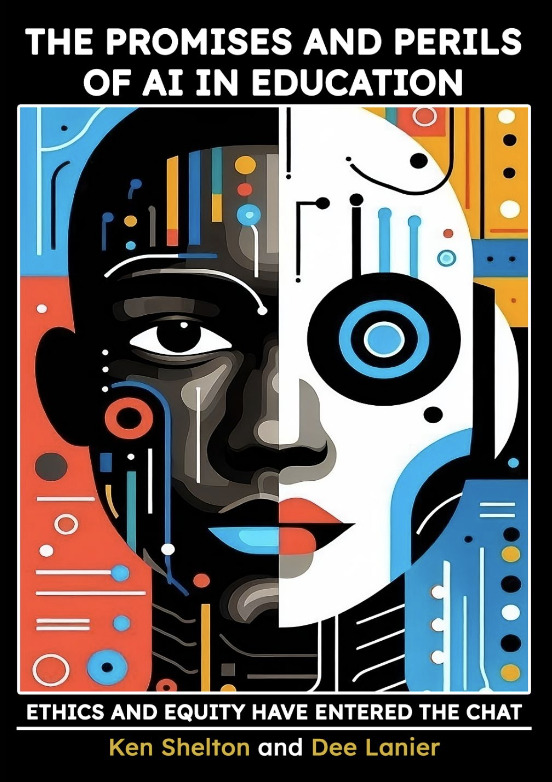
Let’s illustrate this with a simple, interactive exercise both authors utilize use in our AI seminars:
Step 1: Open a search engine. Use any major one you like.
Step 2: Search for “professional hairstyle.” Note the types of images that appear. Pay attention to hair texture, length, styles, and the race/ethnicity of the people modeling those hairstyles.
Step 3: Now, search for “unprofessional hairstyle.” Are there significant differences in what shows up? What assumptions are being reinforced?
After you have completed this simple exercise, reflect on whether your own internal biases could have predicted these results. Then remember, this is the same data that is being used to train our AI systems. The bias that exists “out there” is the same bias that exists in ourselves. Unfortunately, workshop facilitators emphasizing the many advantages of AI frequently offer only superficial or tokenistic advice regarding the identification of biases in AI outputs, rarely providing practical guidance on uncovering or mitigating such biases. Almost to the point of it becoming a comment to be made, but an action to be ignored.
Before evaluating AI, it is essential that we scrutinize ourselves for bias (Am I Right?). Once we’ve reflected critically on our own biases, we can develop skills to question what AI shows us, rather than accepting it outright.
Transparency about training processes is essential as well. As we integrate AI into education, let’s remain alert to biases creeping in. With self-awareness and care, we can leverage AI’s potential while protecting equity. This isn’t about getting the “right” answer as much as realizing how quickly algorithms can mirror our prejudices and societal biases. If an algorithm used for hiring processes is trained on datasets that equate “professionalism” with certain hairstyles, you’ve built a biased system right from the start. This surfaces how algorithms profoundly shape perceptions and cement discriminatory norms.
“Professional” reinforces dominant appearance standards rooted in bias against textured hair. If a company has a history of only hiring males in leadership roles, or promoted men to leadership roles, this pattern of selective bias will be so embedded in the data sets that it will undoubtedly be recursive.
Left unchecked, AI has the potential to amplify the bias that exists in our human psyche. This bias gets coded into the algorithms that power our security systems, impacting performance, posture, and punishment. Before you worry about the potential harms of AI, consider research conducted by the Yale Child Study Center in 2016. Researchers used eye-tracking software and found that educators showed a tendency to more closely observe Black students, especially boys, when expecting challenging behaviors. This “over-policing” is unconscious because our bias suggests Black boys are more likely to cause trouble.
This same baked-in bias gets coded into the software that glances at all children but lingers on Black boys who might be perceived as troublemakers. Then rather than questioning either our own perceptions or that of the algorithm, we simply accept it because “that’s the way we have always done it,” thus perpetuating the harm caused.
Increasing transparency in data sourcing and algorithm development builds trust. Implementing strong oversight, accountability structures, and partnerships with diverse rightsholders is key for ethical AI development and deployment in schools. Curricula should embed critical thinking activities that teach students to question AI recommendations and identify potential biases.
Implementing carefully designed policies and regulations is crucial for ensuring the ethical deployment of artificial intelligence technologies in educational settings. Such policies and regulations must explicitly prohibit and actively counteract all forms of discrimination, including but not limited to racism, ageism, sexism, ableism, classism, and colonialism. These deeply entrenched systemic biases have long permeated societal institutions like education, perpetuating marginalization and oppression of vulnerable groups. As AI systems are not value-neutral but inherently reflect the biases and worldviews of their creators, a failure to institute robust safeguards risks further entrenching and amplifying existing disparities.
We have an obligation to engage in a continuous dialogue of personal and platform interrogation. We urge AI tool developers to foster inclusive development teams that incorporate diverse perspectives from the outset to mitigate bias risks. This includes both cultural diversity and the involvement of individuals most susceptible to the adverse effects of biased outputs and hallucinations. Only when individuals and institutions voice their concerns and wield their consumer power will there be a shift towards fairer access to, and outcomes from, AI.
To address ingrained biases and create educational AI that fulfills its potential while safeguarding vulnerable students, a comprehensive approach is required. This approach should encompass data, algorithms, transparency, accountability, education, and inclusive team building. A proactive approach is required when addressing systemic issues related to bias in educational AI. This involves asking the right questions, gathering the necessary data, and intentionally examining disparities in access, treatment, and outcomes between students of diverse backgrounds.
Passively ignoring recurring issues year after year is not an option. Genuine curiosity paired with a willingness to challenge the status quo are essential for altering harmful patterns. Embracing a collaborative mindset is paramount. Rather than perceiving AI products as impenetrable entities, we should engage with them as conversational partners. By posing critical inquiries, analyzing responses, and utilizing AI-powered insights to foster meaningful discussions, we can collectively discern areas for enhancement.
Gathering diverse perspectives and experiences enriches our viewpoints, making them more holistic. This collaborative approach should be central to the mission of education today. Instead of professional development facilitators simply showcasing the “magic” of AI in schools or employing it as a glorified version of an educator resource marketplace such as Teachers Pay Teachers, educators should be equipped with the skills to empower their students as digital sleuths and advocates for their own rights. As AI strives to become a creative co-pilot and companion for all, let’s ensure we do not neglect the essential groundwork of ethics, social-emotional learning, and equity.
The bias that exists “out there” is the same bias that exists in ourselves.
Ken shelton and dee lanier
On the technical side, bias mitigation techniques like reweighting data samples, augmenting underrepresented classes, and adversarial debiasing during model training can help reduce discrimination risks. However, these must be paired with efforts to improve data collection itself.
Initiatives to gather more diverse, representative training data will reduce dependence on problematic historical sources. Ongoing bias testing using datasets that reflect real-world diversity is also important. Tools that continuously monitor model performance across different subgroups enable rapid detection and iteration on potential issues. In an age where AI promises to transform education, bias remains an insidious threat, potentially exacerbating discrimination against already marginalized students. To mitigate this, we have an obligation to exercise caution about over-relying on technical measures alone.
Even advanced algorithms can perpetuate societal biases, highlighting the equal importance of social and institutional change. Students themselves need to develop analytical abilities to critically assess AI outputs, and diverse AI development teams can help anticipate gaps, deficiencies, design flaws.
Moreover, exploring beyond the most well-known LLMs (such as Open AI’s ChatGPT, Google’s Gemini, and Anthropic’s Claude) opens opportunities for teachers and students to utilize AI that is specifically designed to reduce bias. One notable tool is Latimer.ai, which incorporates books, oral histories, and local archives from underrepresented communities. Founder John Pasmore collaborated with Temple University professor Molefi Kete Asante, an expert in African American and communication studies, to curate this unique dataset.
Developing strong governance frameworks will be critical for ensuring the ethical and fair use of AI in education. Policymakers must enact clear guidelines and regulations around data practices, algorithmic transparency, accountability structures, and anti-discrimination protections. Cross-sector collaboration between policymakers, educators, technology companies, and advocates can incorporate diverse viewpoints into cohesive policies. Government oversight bodies specifically focused on educational AI can conduct ongoing auditing and address emerging concerns.
Teacher training initiatives should include building data literacy and critical technical skills that allow educators to be informed users of AI technologies. Curriculums should also be updated to teach students analytical reasoning abilities and critical perspectives on AI systems. This empowers both teachers and students to identify biases, question recommendations, and push back on problematic outputs while still benefiting from AI’s potential.
Development of free, accessible bias training resources can democratize access to these needed skills. Partnering directly with marginalized communities allows for AI systems to be designed based on real-world diverse experiences and needs, preventing their voices from being excluded. Sustained engagement ensures community priorities are centered ethically. AI developers should commit to transparency about data practices and algorithmic approaches with partners, building trust and shared ownership over technology impacting people’s lives.
Addressing unfair bias requires a comprehensive approach that integrates both technical and social aspects. Through diligence, transparency, and proactive efforts, we can prevent these powerful technologies from inadvertently harming vulnerable youth and instead foster educational AI that advances empowerment and equality. But the work does not end here. Mitigating unfair bias in AI is an ongoing process that requires sustained collaboration between policymakers, educators, technologists, students and families.
It truly is a matter of necessity that we maintain an unwavering commitment to educational equity, continuously evaluate AI systems for discrimination, and demand accountability. With diligence, care, and inclusive ethics guiding development, all students can share AI’s benefits equally, opening doors to personalized instruction and customized support. Our children’s futures depend on the hard work required to get this right. Though the path is challenging, it leads towards just possibilities we have only begun to imagine.
Excerpt from: The Promises and Perils of AI in Education: Ethics and Equity Have Entered the Chat by Ken Shelton and Dee Lanier. Copyright © 2024 by Ken Shelton and Dee Lanier. Published by Lanier Learning, Charlotte, NC. Reprinted by permission of the Publisher. All rights reserved.